Y ou’re at the wheel of your vehicle however you’re tired. Your shoulders begin to droop, your neck starts to sag, your eyelids move down. As your head pitches forward, you swerve off the roadway and speed through a field, crashing into a tree.
However what if your vehicle’s tracking system identified the telltale indications of sleepiness and triggered you to manage the roadway and park rather? The European Commission has actually enacted laws that from this year, brand-new lorries be fitted with systems to capture sidetracked and drowsy chauffeurs to assist avoid mishaps. Now a variety of start-ups are training expert system systems to acknowledge the free gifts in our facial expressions and body movement.
These business are taking an unique method for the field of AI. Rather of recording countless real-life chauffeurs going to sleep and feeding that info into a deep-learning design to “discover” the indications of sleepiness, they’re developing countless phony human avatars to re-enact the drowsy signals.
” Huge information” specifies the field of AI for a factor. To train deep knowing algorithms precisely, the designs require to have a plethora of information points. That produces issues for a job such as identifying an individual going to sleep at the wheel, which would be hard and lengthy to movie occurring in countless automobiles. Rather, business have actually started constructing virtual datasets.
Synthesis AI and Datagen are 2 business utilizing full-body 3D scans, consisting of comprehensive face scans, and movement information recorded by sensing units positioned all over the body, to collect raw information from genuine individuals. This information is fed through algorithms that modify different measurements lot of times over to develop countless 3D representations of people, looking like characters in a computer game, taking part in various behaviours throughout a range of simulations.
When it comes to somebody going to sleep at the wheel, they may movie a human entertainer going to sleep and integrate it with movement capture, 3D animations and other methods utilized to develop computer game and cartoon animations, to construct the preferred simulation. “You can map [the target behaviour] throughout countless various physique, various angles, various lighting, and include irregularity into the motion too,” states Yashar Behzadi, CEO of Synthesis AI.
Utilizing artificial information eliminates a great deal of the messiness of the more conventional method to train deep knowing algorithms. Usually, business would need to collect a large collection of real-life video footage and low-paid employees would meticulously identify each of the clips. These would be fed into the design, which would discover how to acknowledge the behaviours.
The huge cost the artificial information method is that it’s quicker and less expensive by a broad margin. However these business likewise declare it can assist deal with the predisposition that produces a substantial headache for AI designers. It’s well recorded that some AI facial acknowledgment software application is bad at identifying and properly recognizing specific group groups This tends to be since these groups are underrepresented in the training information, suggesting the software application is most likely to misidentify these individuals.
Niharika Jain, a software application engineer and professional in gender and racial predisposition in generative artificial intelligence, highlights the well-known example of Nikon Coolpix’s “blink detection” function, which, since the training information consisted of a bulk of white faces, disproportionately evaluated Asian faces to be blinking. “An excellent driver-monitoring system need to prevent misidentifying members of a particular group as asleep more frequently than others,” she states.
The common reaction to this issue is to collect more information from the underrepresented groups in real-life settings. However business such as Datagen state this is no longer required. The business can just develop more faces from the underrepresented groups, suggesting they’ll comprise a larger percentage of the last dataset. Genuine 3D face scan information from countless individuals is worked up into countless AI composites. “There’s no predisposition baked into the information; you have complete control of the age, gender and ethnic culture of individuals that you’re creating,” states Gil Elbaz, co-founder of Datagen. The scary faces that emerge do not appear like genuine individuals, however the business declares that they’re comparable adequate to teach AI systems how to react to genuine individuals in comparable circumstances.
There is, nevertheless, some argument over whether artificial information can truly get rid of predisposition. Bernease Herman, an information researcher at the University of Washington eScience Institute, states that although artificial information can enhance the toughness of facial acknowledgment designs on underrepresented groups, she does not think that artificial information alone can close the space in between the efficiency on those groups and others. Although the business in some cases release scholastic documents showcasing how their algorithms work, the algorithms themselves are exclusive, so scientists can not individually assess them.
In locations such as virtual truth, in addition to robotics, where 3D mapping is very important, artificial information business argue it might in fact be more suitable to train AI on simulations, particularly as 3D modelling, visual results and video gaming innovations enhance. “It’s just a matter of time up until … you can develop these virtual worlds and train your systems entirely in a simulation,” states Behzadi.
This sort of thinking is making headway in the self-governing car market, where artificial information is ending up being important in teaching self-driving lorries’ AI how to browse the roadway. The conventional method– recording hours of driving video footage and feeding this into a deep knowing design– sufficed to get automobiles reasonably proficient at browsing roadways. However the concern vexing the market is how to get automobiles to dependably manage what are referred to as “edge cases”— occasions that are uncommon enough that they do not appear much in countless hours of training information. For instance, a kid or pet dog facing the roadway, made complex roadworks or perhaps some traffic cones positioned in an unforeseen position, which sufficed to stump a driverless Waymo car in Arizona in 2021.
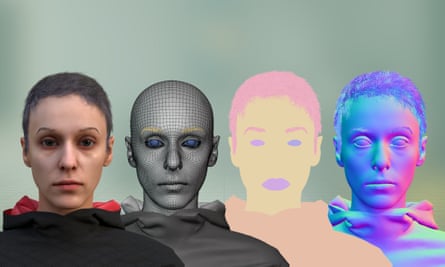
With artificial information, business can develop limitless variations of circumstances in virtual worlds that hardly ever take place in the real life. “Rather of waiting millions more miles to collect more examples, they can synthetically produce as lots of examples as they require of the edge case for training and screening,” states Phil Koopman, associate teacher in electrical and computer system engineering at Carnegie Mellon University.
AV business such as Waymo, Cruise and Wayve are progressively counting on real-life information integrated with simulated driving in virtual worlds. Waymo has actually produced a simulated world utilizing AI and sensing unit information gathered from its self-driving lorries, total with synthetic raindrops and solar glare. It utilizes this to train lorries on regular driving circumstances, in addition to the harder edge cases. In 2021, Waymo informed the Brink that it had simulated 15bn miles of driving, versus a simple 20m miles of genuine driving.
An included advantage to screening self-governing lorries out in virtual worlds initially is reducing the possibility of extremely genuine mishaps. “A big factor self-driving is at the leading edge of a great deal of the artificial information things is fault tolerance,” states Herman. “A self-driving vehicle slipping up 1% of the time, or perhaps 0.01% of the time, is most likely excessive.”
In 2017, Volvo’s self-driving innovation, which had actually been taught how to react to big North American animals such as deer, was baffled when experiencing kangaroos for the very first time in Australia. “If a simulator does not understand about kangaroos, no quantity of simulation will develop one up until it is seen in screening and designers determine how to include it,” states Koopman. For Aaron Roth, teacher of computer system and cognitive science at the University of Pennsylvania, the difficulty will be to develop artificial information that is identical from genuine information. He believes it is possible that we’re at that point for face information, as computer systems can now produce photorealistic pictures of faces. “However for a great deal of other things,”– which might or might not consist of kangaroos– “I do not believe that we exist yet.”